Summary
An important challenge in building a quantum computer is quantifying the level of control obtained in the preparation of a quantum state. The state of a quantum device is characterized from experimental measurements, using a procedure known as tomography. Exact tomography requires a vast amount of computer resources, making it prohibitive for quantum devices larger than a few qubits. In this project we develop a practical, approximate tomography method using modern machine learning techniques. Our work is based on training artificial neural networks using measurement data obtained from a system of qubits. After training, the neural network is sampled to determine properties of the underlying quantum state. As part of a collaborative effort, we will demonstrate our machine learning algorithms on both synthetic and experimental measurement data. Our ultimate goal is to deliver practical machine learning technology to design and characterize near-term quantum devices.
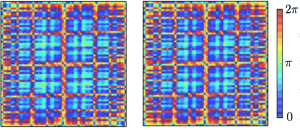
Figure 1. A visualization of the phases of a quantum wavefunction of 100 qubits. At left, the exact value of the phases, obtained from a large-scale computer simulation. At right, the phases reconstructed with state tomography using artificial neural networks.
Related Content
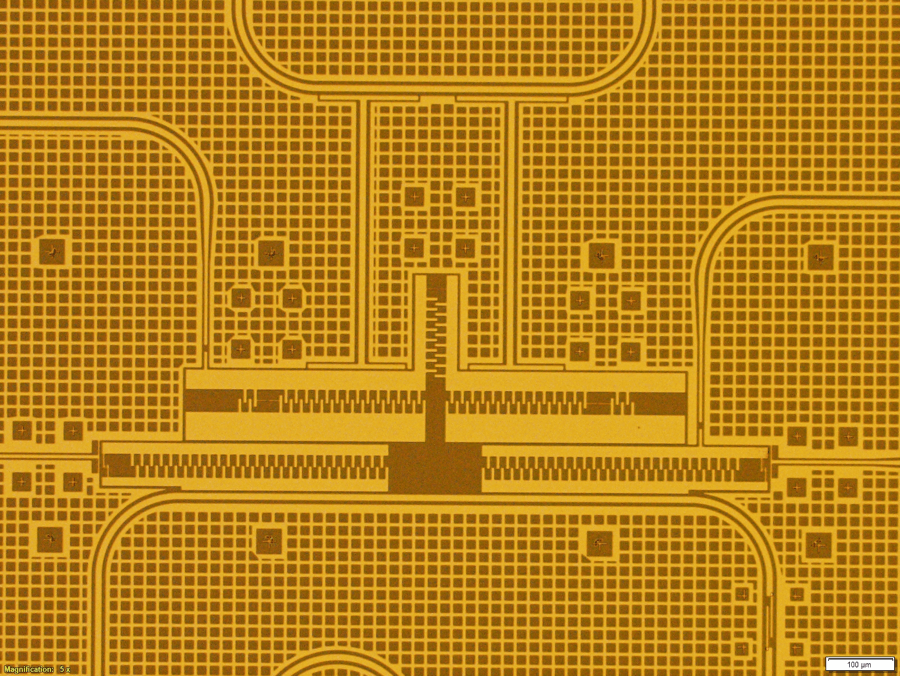
Quantum Simulation of Strongly Coupled Field Theories
Strongly-coupled field theories describe both fundamental and applied quantum problems.
August 10, 2017
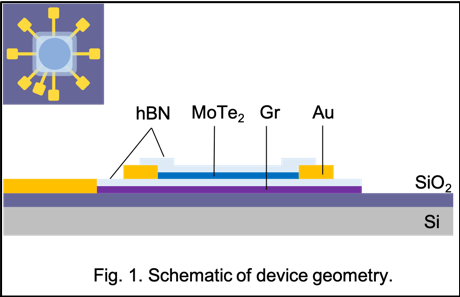
Combined momentum- and real-space photoelectric probes of dimensionality-tuned Weyl semimetals
Summary The library of two-dimensional (2D) materials has recently grown to include topological insulators and semimetals. Their incorporation in special device geometries may lead to novel quantum electronics with enhanced functionalities. Weyl semimetals, in particular, offer the most robust form of topological protection. Recent results from our group indicate that Weyl nodes should be […]
March 12, 2019
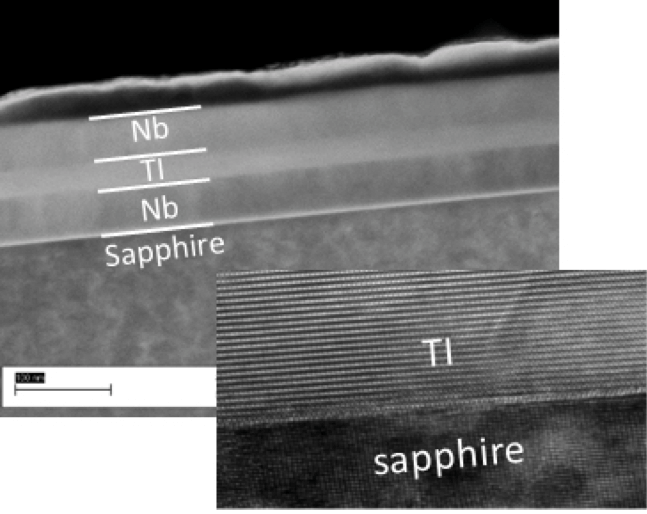
Hybrid Quantum Materials towards Topological Quantum Computing
Summary Proximity engineered hybrid materials have shown promise for topological quantum information processing. This form of quantum computing provides a stable, error-tolerant approach for building scalable quantum information processors. Topological quantum computing relies on braiding non-Abelian particles, such as Majorana fermions, which do not exist in nature. One can however use materials engineering to […]
December 8, 2018
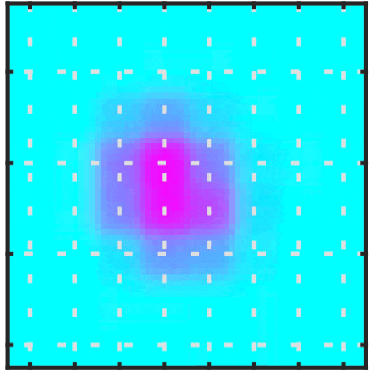
Novel High-Speed Receiver for Quantum Communication and Sensing
Summary An essential aspect of a quantum channel is the detection and analysis of quantum signals in the form of photons. For most free-space applications, the photons are polarization encoded, e.g. by assigning the ‘0’ to horizontally polarized photons and ‘1’ to vertically polarized photons. However, where the geometric reference is not constant at all […]
January 1, 2019