Summary
The main protease (Mpro) in severe acute respiratory syndrome coronavirus 2 (SARS-CoV-2), the virus responsible for the coronavirus disease (COVID-19), has emerged as a promising drug target. The scientific community has produced a large number of crystallographic structures of the protease, which mediates viral replication and transcription. These structures report several fragments with varied chemotypes binding to different sites in Mpro. The main challenge at this stage is to effectively corroborate these valuable structural insights and expedite the search for any known drugs or natural products with properties similar to those fragments such that they can be rapidly translated for clinical testing against SARS-CoV-2. In this project, we build an artificial intelligence-based model using the available structural data of fragment-bound SARS-CoV-2 Mprocomplexes.Leveraging known drug-target interactions, our goal is to produce a machine learning algorithm capable of predicting potential drugs that can be repurposed for the treatment of COVID-19. We explore the potentials of small molecules, including drugs, natural products, and quantum dots to identify promising structures for inhibiting and/or detecting the SARS-CoV2 virus. Our results are expected to provide useful insights into the readily available therapeutic resources and help in the fight against the COVID-19 pandemic.
Related Content
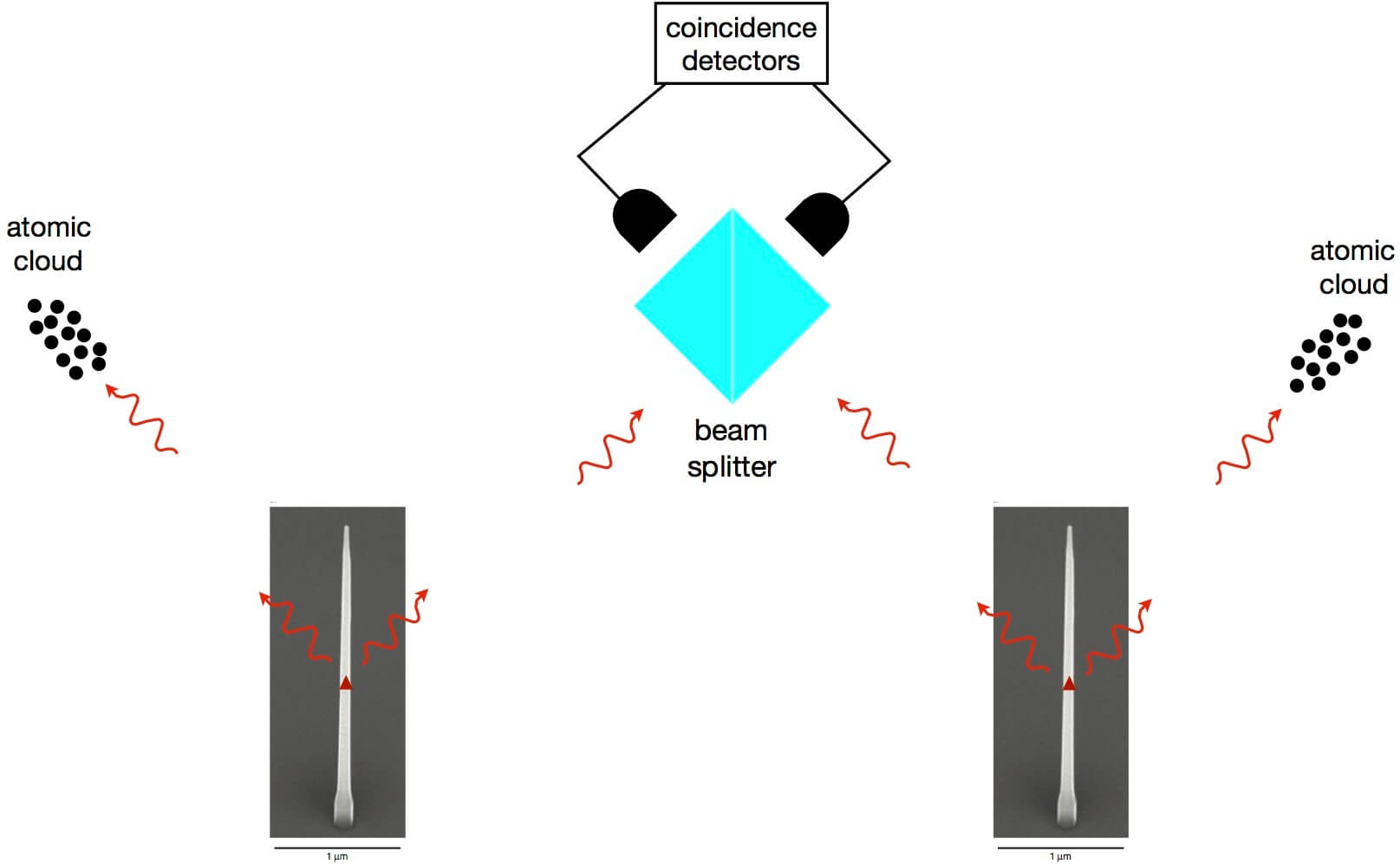
Hybrid Quantum Repeater based on Atomic Quantum Memories and Telecom Wavelength Entangled Photon-Pairs Generated from Semiconductor Nanowires
Summary Losses in physical channels, such as optical fibres, limit existing quantum communication systems to modest distance ranges. Since amplification of quantum signals is fundamentally not possible, we look to extend the range and functionality of these quantum channels by adding quantum memory nodes that can daisy-chain multiple lengths of quantum channels through entanglement […]
October 29, 2018
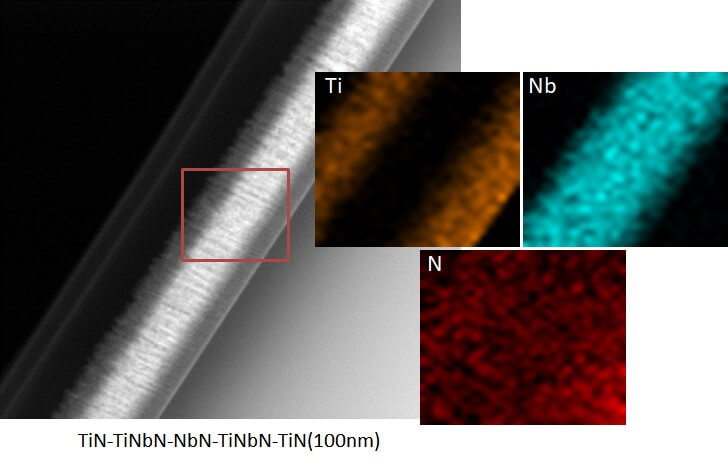
Composite Superconductors for Improved Quantum Coherence
Summary Conventional superconductors have trouble performing well in magnetic fields required for electron spin resonance (ESR) – based quantum information processing applications. We can, however, use proximity engineering to select desired properties from different materials and combine them for improved superconducting performance in magnetic fields — an improvement that would have strong implications for […]
December 12, 2018
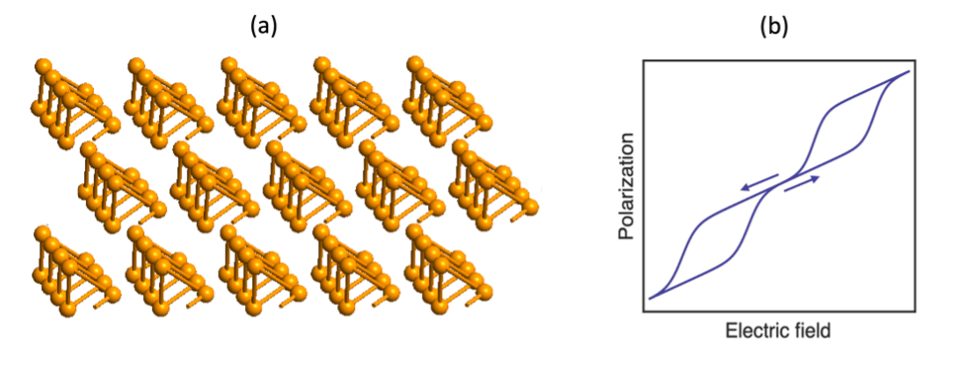
Enabling Next-Generation Sustainable Computing through Novel Multi-Valued-Logic Quantum Devices
As the demand for digital services grows, so does the need for data centres and transmission networks. Unfortunately, these data systems consume vast amounts of energy, resulting in nearly 1% of all energy-related greenhouse gas emissions. This project aims to invent novel quantum devices for highly energy-efficient computing that may help reduce the global digital […]
June 12, 2023
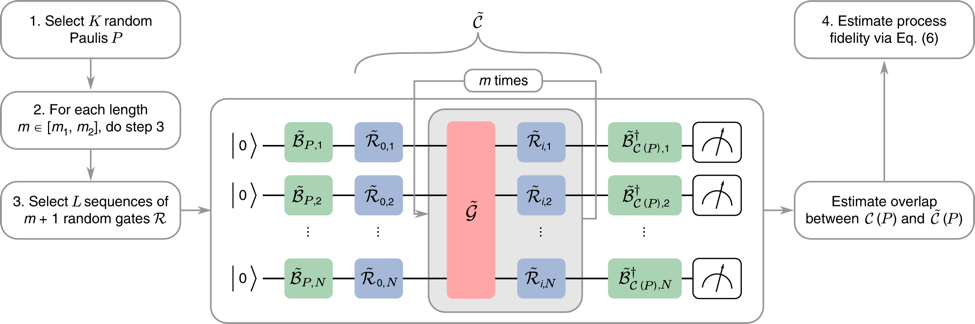
Reliably operating noisy quantum computers
Summary The overall goal of the project is to develop practical methods to be able to reliably run useful applications on near-term quantum computers. This requires identifying and overcoming the ubiquitous errors that currently limit quantum computing capabilities. Traditional methods of quantifying errors in quantum computers fail to predict how errors affect the output of […]
January 22, 2020