A brain-inspired iontronic platform that mimics hardware-based artificial intelligence
Wednesday, August 7, 2024
Researchers supported by Transformative Quantum Technologies (TQT) have demonstrated an iontronic platform that is configurable to mimic neuromorphic functions on a hardware level.
With the rise of artificial intelligence (AI), Internet of Things (IoT), and big data technologies, coupled with the limitations posed by the von Neumann architecture, neuromorphic computing architectures inspired by the human brain have been developed. Traditionally, in the von Neumann architecture, the memory and processor are physically segregated, causing latency and higher energy consumption due to sequential data traffic. In contrast, non-von Neumann architectures, such as quantum computing and neuromorphic computing (NC), feature high degrees of parallelism, where memory and processing elements are co-located. The co-location enables the machine to “think” in concepts instead of following instructions in a sequential manner.
Among various hardware platforms for artificial neural network (ANN), memristor-based ANN is the most attractive because memristors can respond to external stimuli with “memories”. In other words, their response to the external stimulus can be carefully “trained”. In typical analog-type memristor devices, conductance can be gradually tuned by applied voltage or current, mimicking biological synapses. Further inspiration originates from neuron functionalities, where the regulated injection, transport, capture and release of ions throughout biological channels are essential to information processing or electrical triggering of physical activities. Instead of Na, K and Ca ions which normally function in aqueous biological systems, lithium ions are known for faster and more efficient transport in fully solid-state systems, making them more suitable for larger-scale integrations.
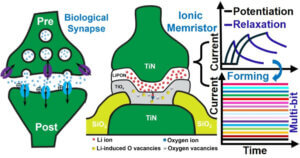
Figure 1. Here, the yellow spheres represent Li-ion-induced vacancies, and the gray ones are native oxygen vacancies already in TiOx. Li ions preferably combine with oxygen and chemically reduce Ti, which has similar effects as forming oxygen defects in TiOx (reducing x). Therefore, the Li-ion insertion/extraction increases/decreases the device conductivity accordingly.
Guo-Xing Miao (Associate Professor, the Department of Electrical and Computer Engineering), Manoj Sachdev (Professor, Department of Electrical and Computer Engineering), and their team demonstrated a Lithium-ion imbued TiOx iontronic device with programmable volatile and non-volatile memory capabilities. The device conductance exhibits synapse-like short-term plasticity (STP) with controlled ion injection (under stimulating electrical pulses of desired strengths and durations) and subsequent spontaneous retraction. The ion relaxation can be accelerated with an opposite electrical bias for assisted extraction if an application demands faster time scales. After a forming process which soft breaks down the device with a conducting channel, the device can effectively retain long-term memories analogously or in the digital form of multibit per unit. Hence, on the same platform, devices can be configured as volatile units, which can be used as non-linear reservoirs for neuromorphic training, or be configured as non-volatile units serving as the storage layer. This creates a universal platform that can be custom-programmed for applications on an as-needed basis.
“We finally have a true iontronic device, inspired by both renewable energy and brain functions where the roles of ions are already crucial,” said Miao. “We demonstrated a practical and CMOS compatible platform that utilizes a “battery-like” structure for more energy-efficient information processing.” As a demonstration of principle, by applying both tunable volatile and non-volatile versions of the devices to a reservoir computing architecture, the team simulated voice recognition tasks with an accuracy of over 94%. The team’s goal is to demonstrate a customizable CMOS chip that can perform energy-efficient, in-memory neuromorphic computing – a hardware realization of artificial brains.
“The devices’ back end of line (BEOL) integration onto CMOS chips has also been achieved and a larger scale demo is underway. This has further implications for exotic quantum materials, such as many 2D materials and superconductors, strictly forbidden in foundries, to be integrated with CMOS technologies in the very near future,” explained Miao.
Miao expects this research to become the milestone for a power-efficient non-von-Neumann platform for hardware AI. It also has FPGA-like programmability making it highly versatile in adapting to new applications or algorithms.
Volatile and Non-volatile Programmable Iontronic Memristor with Lithium Imbued TiOx for Neuromorphic Computing Applications was published in ACS Nano by Rabiul Islam, Yu Shi, Gabriel Vinicius de Oliveira Silva, Manoj Sachdev, and Guo-Xing Miao.
This project is supported in part by the Canada First Research Excellence Fund through the Transformative Quantum Technologies (TQT) program.